Blog
The importance of data segmentation for enterprise brands
Published on February 13, 2025
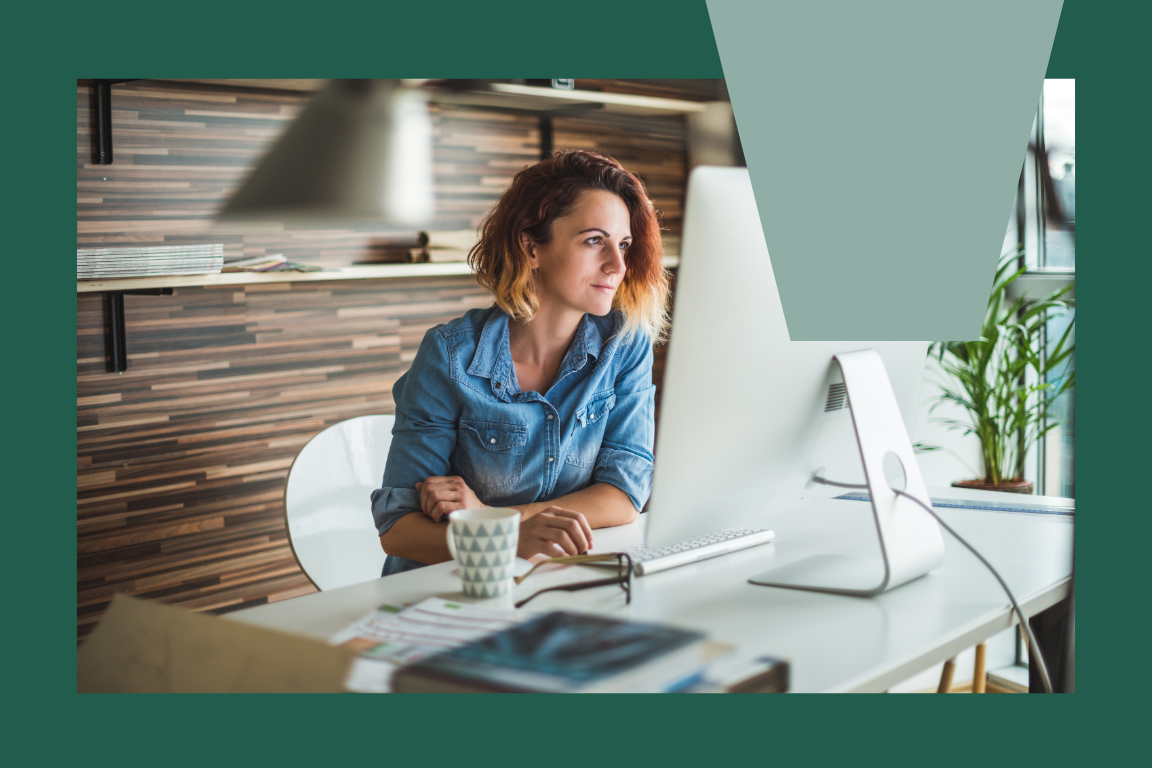
Enterprise brands are drowning in data. Every customer interaction, transaction, and digital footprint generates terabytes of information – a goldmine for growth, but only if you can extract actionable insights from the noise.
Raw data is just that – raw. Without strategic organization, even the most advanced enterprise brands struggle to translate this deluge into meaningful business outcomes.
Data segmentation is the catalyst for turning this chaos into clarity. For enterprise brands, it’s the backbone of personalization at scale, operational agility, and customer-centric innovation. Yet, many still treat it as an afterthought, leaning on outdated, siloed datasets that fail to reflect real-world complexity.
Read on to explore how data segmentation transforms fragmented insights, why legacy approaches fail, and how modern architectures are bridging the gap.
What is data segmentation?
Data segmentation is the process of organizing unstructured datasets into smaller, logically grouped subsets. While closely related to audience segmentation, the two concepts differ in scope:
- Audience segmentation focuses on individuals, grouping them based on shared traits like demographics, behavior, or preferences.
- Data segmentation is broader and applies to any type of structured or unstructured data, including transactional records, inventory data, or geographic trends.
For example, segmenting sales data by region is data segmentation; grouping customers by purchase history is audience segmentation. Both organize information but differ in application.
Key segmentation types
While tactics vary by industry, enterprise brands typically segment data across five core dimensions:
- Demographic: Age, income, job title. E.g. luxury automakers targeting C-suite execs
- Behavioral: Purchase history, app usage patterns. E.g. SaaS companies tracking feature adoption rates
- Geographic: Regional preferences, climate-driven demand. E.g. retailers adjusting inventory for seasonal shifts
- Psychographic: Values, lifestyle, beliefs. E.g. eco-conscious segments for sustainable product launches
- Firmographic (B2B): Company size, industry, revenue. E.g. software vendors targeting Fortune 500 accounts
These categories help organizations tailor strategies across departments, all the way from marketing to supply chain management.
Data segmentation and the warehouse advantage
At enterprise scale, advanced data segmentation is a strategic imperative. By leveraging tools that integrate directly with your cloud data warehouse, you can unlock precision, agility, and governance at a fraction of the cost and complexity of legacy systems.
Precision targeting and personalization
Data segmentation fuels hyper-relevant customer experiences. Whether it’s tailoring email campaigns by purchase history or serving dynamic ads based on real-time browsing behavior, granular segments (like cart abandoners or loyalty members nearing renewal) make sure every touchpoint resonates.
Leveraging live insights from your data warehouse means behavioral signals – app interactions, POS transactions, loyalty activity – always reflect the freshest data. For example, triggering a win-back offer the moment a high-value customer’s engagement dips – without third-party CDP dependencies or stale exports.
Cost and operational efficiency
Segmentation reduces wasted spend by zeroing in on high-impact audiences. That means excluding low-intent users from paid ads, focusing retention efforts on high-CLV customers, and suppressing recent buyers from redundant promotions. Every campaign is sharper, so every dollar works harder.
Plus, when segments are built directly from your centralized data cloud, you can retire costly, redundant tools (bye, standalone CDPs) and reduce engineering bottlenecks. With a low-code visual segmentation interface, marketing teams can build audiences themselves and launch campaigns faster – without waiting on devs for SQL queries or sluggish ETL jobs.
Agility and future readiness
AI-powered segmentation flips reactive marketing into proactive execution. Machine learning models trained on unified datasets can auto-generate predictive segments – e.g. churn risks, upsell targets – while reverse ETL activates these insights across channels without custom pipelines or system silos. This agility lets teams deploy AI-driven segments in hours, not weeks, and pivot in-flight campaigns the second trends shift.
Cross-functional alignment
Segmentation breaks down silos by creating shared audience definitions that can be used consistently across teams.
When connected directly to your warehouse, you can establish a single source of truth. The high-value customer segment driving email campaigns is the same segment informing finance’s CLV models and product’s feature adoption analysis. This alignment eliminates conflicting definitions, reduces engineering requests, and guarantees every campaign ladders up to company-wide KPIs.
Compliance and governance
Warehouse-native tools automate governance by enforcing rules at the source and dynamically excluding users who opt out or fall under GDPR/CCPA. Consent flags and do not sell lists stored in the warehouse filter every segment, meaning campaigns adhere to global regulations by design – no manual list uploads or risky data exports. Compliance becomes audit-ready, embedded in your workflow from the ground up.
The challenges of data segmentation
Data silos and fragmented systems
Legacy martech stacks scatter data across CDPs, CRMs, and messaging platforms, forcing teams to duplicate, sync, and constantly reconcile inconsistencies. One system says a customer is active; another says they churned. The result? Stale segments, mismatched counts, and frustration all around.
Connecting directly to your warehouse collapses these silos and unifies first-party data – without replication. Every team across the org works from the same live datasets. When a customer moves from active to high CLV, that shift reflects instantly across campaigns, reports, and strategies. No more reconciling conflicting numbers between platforms.
Slow, manual segmentation workflows
Marketing ops often wait days, even weeks, for engineering to build ETL pipelines, export CSV lists, or update segment criteria. Tools with native warehouse integration put control back in the hands of non-technical teams. For example, a loyalty manager can refresh a lapsing members segment and push it to downstream tools without engineering tickets or CSV handoffs.
Costly, redundant tools
Overlapping martech tools drain budgets and create unnecessary complexity. Custom pipelines to connect all of your various martech tools pile on costs for duplicative data storage and usage while simultaneously locking teams into stale data. By retiring expensive legacy tools and using a warehouse-native approach, you can reduce engineering debt while improving campaign performance – without the bloat.
Lagging real-time action
Batch-based segmentation slows everything down. Campaigns get delayed by hours (or days), causing brands to miss prime engagement windows. That means cart abandoners slipping away before a sale ends, or worse, customers getting “Come back and buy” emails after they’ve already checked out.
With access to real-time behavioral data, segments update continuously. A customer exits your site? Trigger an email, mobile push, or ad workflow instantly. They convert before the message sends? Remove them automatically – no awkward or mistimed nudges.
Limited scalability
Legacy tools struggle with enterprise-scale data. Millions of customers, billions of events – most platforms can’t keep up, forcing teams to use subsets of data or simplify segments just to make it work.
Warehouse-native tools process massive datasets directly, so brands can segment millions of customers with unlimited attributes using scalable SQL jobs – without row limits or performance bottlenecks.
Drive growth with warehouse-native data segmentation
Data is the lifeblood of enterprise growth. For martech leaders grappling with fragmented tools, manual workflows, and cross-functional chaos, the solution lies in leveraging your data warehouse as a command center – not just a storage unit.
By eliminating silos, reducing reliance on costly legacy systems, and unlocking real-time insights, warehouse-native segmentation transforms raw data into a precision engine for growth. It’s how you cut costs without sacrificing performance, future-proof for AI-driven demands, and finally align cross-functional teams around the same source of truth.
Your next move
Ready to replace martech complexity with the full power of your data warehouse? MessageGears is the only platform engineered for enterprise-scale segmentation and activation – directly from your cloud data warehouse.
Leading enterprise brands use MessageGears to:
- Launch hyper-targeted campaigns in minutes using live data – no replication or delays
- Cut costs by eliminating redundant CDPs, ESPs, and engineering dependencies
- Scale AI-driven strategies with native machine-learning integration
- Govern data with confidence, embedding compliance into every segment and campaign
The future belongs to brands that harness their warehouse as a growth engine. Schedule a demo with MessageGears today and see how leading enterprise brands are redefining segmentation – without rebuilding their tech stack.